Table of Contents
Introduction to probability axos
As the world grows more complex and populated with information, the need to manage uncertainty has emerged in the form of probability models and probability axos. The ability to forecast market prospects and value risks across various sectors is astounding. However, placing blind trust in forecasts that attempt to harness the most advanced algorithms constructed by human intellect is filled with danger.
Diving into the depths of probability axos, it’s clear that a balance is needed where human judgment must be incorporated to make decisions, and reason must take place alongside intuition. Explore with us as we uncover the unbiased truth behind analytics’ endless possibilities.
The Limitations of Predictive Analytics and Probability Axos
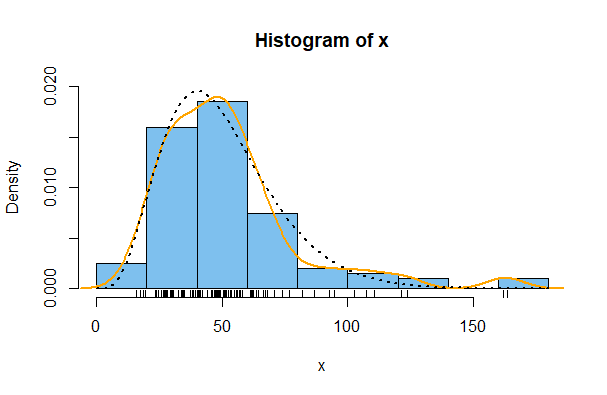
Like most things, predictive analytics has its pros and cons. One challenge includes maintaining data quality. Data that is either biased or flawed renders the model untrustworthy. Another problem is overfitting, which is making a model too complex to capture noise as trends instead of the defining essence. This results in inaccurate real-world application forecasts. Market shifts and new, unprecedented events can be challenging when relying on historical data.
These changes render past trends obsolete. Quantifying social factors is a challenge and plays a significant role in decision-making, which makes it rather difficult for algorithms to account for them.
These days, with the flexible demand placed on analysis and strategy formulation, gaps in realizable insights reveal that great adaptability to new information or shifting conditions can go unprioritized.
Factors that Can Affect Predictions of Probability Axos
So many factors can significantly impact reliance on the probability axis’s forecasts.
The quality of the data is crucial. If the data to be analyzed is corrupted, old, or prejudiced to an extent, it will produce results similarly. This can result in inadequate, misleading choices based on wrong predictions.
Market volatility plays a critical role as well. Sudden modifications in purchase intentions or unforeseen economic turns can make previous models useless in a few days. Don’t neglect human bias either. Models could be biased in the way that analysts inadvertently add their perceptions, which alter calculated results to give a skewed reality based on preconceived ideas instead of observable facts. Moreover, predictive abilities are changed all the time because of new technologies. Changes in algorithms and new machine learning methods are constantly being invented, which leads to how data is analyzed and trends are interpreted. Changes in the outside world, such as geopolitical problems or natural catastrophes, can significantly disturb the predictable models based on probability, customarily used for accurate predictions.
Case Studies: Examples of Failed Predictive Models and probability axis
A probability axos failed example of a model is “Google Flu Trends”—a clear case of model failure. The program aimed to estimate flu outbreak frequencies using search queries, but it initially seemed to work. It later became clear, however, that it was overestimating the relative activity of flu symptoms.
Target has also had its fair share of blunders, having once predicted a teenage boxer’s pregnancy before her family was even aware. They did, of course, have to deal with the backlash of being right about anticipating prison sentence maternity clients, which was ethical negligence due to privacy violations.
The financial sector isn’t off the hook, either. Complex models attempting to project the economic 2008 collapse solely through historical data faced brutal reality when using illusions of stability with other variables. The predictions suffered greatly when unforeseen factors were introduced. These global examples show how predicting probabilities with equally sophisticated tackle systems while lacking basic considerations of human elements and unpredictable situations can easily lead organizations astray.
The Importance of Human Interpretation and Input of Probability Axos
A blend of algorithms and data has always been analyzed using myriad heuristics, attempting to make predictive models. However, the strategies lack the subtle nuance that only the convergence of human insight brings, allowing for a truly unique outlook. Deductive reasoning harnesses the latent predictive potential woven intricately into the data fabric.
Since models can provide, probability axos humans possess the imaginative ability to connect the dots, place them in context, and redraw decisions along with their possibilities. This also brings intuition to a new height, where the algorithm blindly assumes things.
Beneath the surface of raw data, humans can interpret and add many unseen factors that data might sideline—putting a name to things often overlooked aids in reducing errors concerning data omission. Human imagination can pull forth surreal accuracies with the scattered model data along the possibilities. Additional viewpoints deepen interpretive streams, which allow them to sculpt advanced sets. Having teams deliberate on comprehensive outcomes allows the devised strategies to bolster the chances of success.
Furthermore, probability axos assumption reliance is countered with sobering logic when humans step into play. With a productive form and sane exploration of labeling given scenarios through constant questioning and backed reasoning, models can be honestly questioned. Attributing solely to mortals to devise values protected behind statistics alone bonds a layer of safety to any envisioned predictive output.
Implementing a human touch enables constant flexibility to change in the environment. Strategies anchored on statistics change with fluctuating signal intercepts, whereas blending numerical addition with emotional input guarantees steadiness. Stacking analytical judgment and human twists fosters easier results with flexibility built in, cutting through navigating strife entwined with uncertainty crafted as a result.
Using a Combination of Data and Intuition for Better Decision-Making
A decision can be made from the available information, but intuition offers a vital layer. Historical and predictive analyses provide insight into the outcomes of certain events, but the human aspect is frequently disregarded. Intuition is the combination of knowledge and a “gut feeling.” Merging these two with data assists in pointing out the details that are commonly ignored. Such astounding combinations lead to excellent problem-solving strategies.
Picture a business that is adjusting its strategies to accommodate shifts in the market. The data provides a predefined suggestion, unlike the rest of the team, who have a hunch that is equally valuable.
Ignoring their intuition could turn out to be disastrous. With calculated risks, these combinations can deliver astonishing results. Companies whose leaders adapt and accept change build more proficient teams and strategies. Without becoming overly dependent on statistics or trends, it probability axos enables them to navigate uncertainty boldly. This dual approach results in organizational alignment and boosts engagement at all levels.
Conclusion: The Need for a Balanced Approach to Probability Analysis
Statistically driven solutions miss the mark when it comes to the very subtle yet essential details that are captured through experience. Organizations justify their actions based on data, but applying skills associated with data-driven decision making takes it to a whole new level. With the level of human intelligence and machine analytics mastery, the focus is on capturing the benefits brought about by the strengths of statistics and judgment. Employing both approaches gives a more profound understanding of the underlying view towards risks and opportunities exposed by uncertainties. Working believing this ideal enables proper symmetry, allowing confident navigation through the multi-dimensional world.